Google’s Achilles' Heel and Zero-Cost Airdrop Opportunities
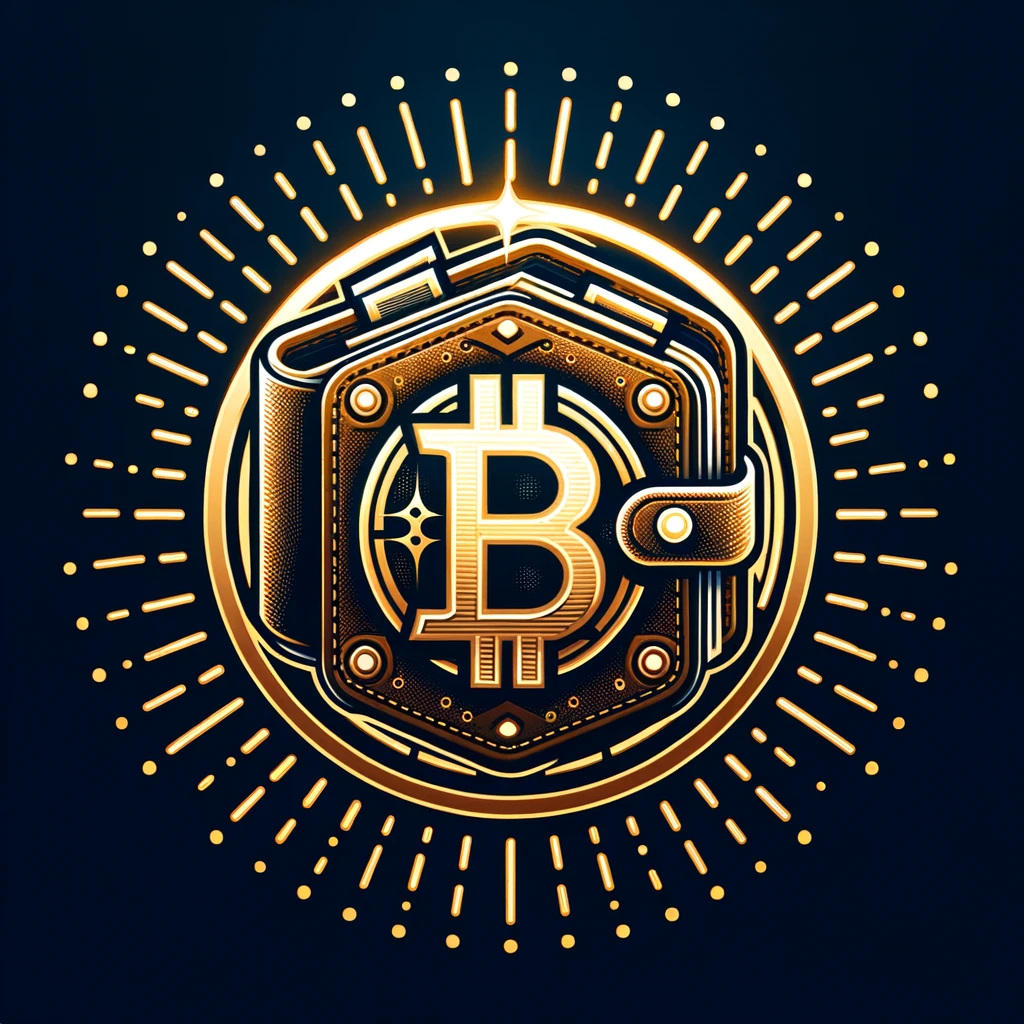
This article was originally published in Chinese on May 29, 2024. For more details, click here.
By opening this article, you already have three zero-investment airdrop opportunities. Read patiently, and you will gain even more.
People have long complained about Baidu, but we don't seem to have many bad impressions about Google.
Although there was a critical error when Bard, a competitor to ChatGPT, was launched in February 2023, causing a significant stock price drop and a temporary $200 billion loss in market value, Google seems unscathed when looking at the latest stock price trends.
Since its IPO in 2004, Google's position as the leading search engine has remained unchallenged, even after exiting the Chinese market.
Many believe Microsoft’s Bing, empowered by ChatGPT, might surpass Google.
However, Google just shook a little.
Because Google’s first-mover advantage is incredibly strong.
Google has long accumulated vast amounts of data and high-quality search indexes, giving it a significant edge in providing accurate and relevant search results. Google processes over 3.5 billion search requests daily, providing its search algorithms with ample training data and optimization opportunities.
Take Google’s Knowledge Graph, for example. It includes billions of entities and hundreds of billions of facts, enabling Google to offer more accurate and detailed information when handling complex queries. For instance, when users search for a historical event, Google not only provides relevant web links but also displays a timeline, key figures, and related images directly on the search results page. While Bing offers similar features, its data scale and quality are not on par with Google.
Additionally, Google has its large language models: PaLM (Pathways Language Model) and Bard.
PaLM, based on the Pathways architecture, excels in multitask learning and efficient computing, capable of handling tasks like language translation, text generation, and question-answering systems. It enhances the accuracy of search results and improves the performance of intelligent dialogue systems like Google Assistant.
Bard focuses on generating and understanding complex long-form content, suitable for writing assistance and content creation. It excels in processing technical documents and legal texts and can generate multimodal content, including images and videos. Bard is widely used in education and training, helping generate detailed teaching materials and training manuals.
Through PaLM and Bard, Google maintains its leading position in the field of large language models, enhancing user experience and driving the development of natural language processing technology, thereby improving the quality of search results.
Of course, Bing, boosted by ChatGPT, is not without gains. The gap in market share between Bing and Google is narrowing. In 2021, Google held about 92% of the global search market, while Bing had less than 3%. By 2023, Bing’s market share had risen to 10%.
In short, although ChatGPT is currently the most advanced large language model, it has only raised Bing’s market share to 10%.
So, can Google rest easy? No. Google not only cannot rest easy but is also caught in the innovator’s dilemma.
The concept of the "innovator's dilemma," proposed by Clayton Christensen in his 1997 book The Innovator's Dilemma, explains why leading companies often lose their market leadership during technological changes due to focusing on existing customers and business models while ignoring disruptive innovations.
Generative AI, represented by ChatGPT, is a disruptive innovation of this era, and Google has similar technologies. We briefly analyzed that it affects Google but is not fatal.
Of course, there are many new technologies in this era, not as easily accepted as ChatGPT, and whether they are disruptive remains to be seen.
Let’s shift our thinking and analyze what might make Google lose its market leadership, i.e., Google’s Achilles' heel. This will help us discover opportunities for newcomers. Naturally, the dilemmas faced by web 2.0 search giants are opportunities in the web 3.0 era.
So, what is Google’s Achilles' heel?
Google’s Achilles' heel lies in superficial information overload and inherent indexing insufficiency.
1. Information Overload: The Most Prominent Issue for Google Users
Information overload is a problem for all web 2.0 search engines, including Google, Bing, and Baidu.
What is information overload?
In a nutshell, every time you search, you may get millions or even billions of web pages.
Statistics show that the average search query results in over 50,000 pages, but users typically only look at the first few pages. This means that most information is ineffective for users, who must spend a lot of time filtering and extracting useful information.
For example, you search for the most popular ETH ETF in the crypto community. Google’s first-screen result looks like this: the first answer is a webpage discussing three questions about the sudden approval of the ETH ETF by the SEC. To know more, you must click on each link.
However, there is a search engine that provides different results. It looks like this:
This is Adot, a decentralized AI search engine designed to tackle information overload.
2. How Does Adot Solve Information Overload?
As you can see, Adot uses AI to organize and summarize large amounts of disordered information.
When you search for “ETH ETF,” Adot not only shows relevant approval information but also includes price trends, features, advantages, and risks of the Ethereum ETF. Moreover, each piece of content provides a link for verification. It’s like walking into a restaurant where you can see the menu and watch the chef cook, greatly enhancing the user experience.
This is not all. The next screen in Adot’s search results is an analysis of ETH.
It’s like a mini-encyclopedia, not particularly impressive but quite considerate. What’s even better is that using Adot earns you points, which can be exchanged for future airdrops. This is something Google does not offer.
The specific reward rules are: daily check-in rewards 5 gems, daily search rewards 5 gems, and referring a user rewards 50 gems.
In my experience, Adot is better at searching for blockchain and cryptocurrency-related content. (The operation is straightforward, so I won’t write a tutorial. If you have a Metamask wallet, click on the Adot link and confirm all the way.)
In essence, rewards and AI processing are things Google could do, but it either chooses not to or simply cannot. We will explain this in detail later. Now, let’s continue with the more critical issue for Google—indexing insufficiency.
If information overload can still be tolerated, the lack of proper indexing will completely ruin Google's future.
Google’s indexed data is just the tip of the iceberg.
3. Indexing Insufficiency: The Core Problem Affecting Google's Dominance
No one knows exactly how many web pages Google indexes. However, once you understand the existence of the deep web, you won’t be surprised by the following statement.
Rumor has it that Google only indexes 0.03% of the internet’s data, which might be an exaggeration, but indexing less than 10% is not unfair to Google.
Do you know what this means?
It means your search results are far from complete, with vast amounts of information ignored, especially deep web content.
What is the deep web?
The deep web refers to content not indexed by traditional search engines, including academic research, specialized databases, and corporate intranets, unlike the illegal dark web. This information is often extremely valuable.
For instance, a medical student once needed to find the latest research results on a rare disease. His Google search results mostly consisted of news articles and basic introductions, with no in-depth research data. Eventually, he found the necessary research papers through his school’s internal database—these papers belonged to the deep web, not indexed by Google. If Google could index these deep web resources, users would more easily find high-quality information they need.
Similarly, when companies conduct market analysis, they may need to access detailed financial reports and market strategies of competitors in restricted databases. These are typically hidden behind permissions and are part of the deep web. Analysts have to spend a lot of time on different databases and platforms to find data. If Google could index these resources, it would significantly improve data acquisition efficiency and accuracy.
For a personal experience example, even Baidu cannot collect the content of your WeChat Moments. Not just WeChat Moments, but all the data in the image below cannot be accessed by Google or Baidu. Only WeChat’s search feature can. For other search engines, this data is part of the deep web.
The problem of indexing insufficiency not only wastes users’ time but also limits information access and utilization. For those who need in-depth research and accurate data, this is a significant bottleneck.
Google cannot solve the problem of data indexing insufficiency through improved indexing technology. Moreover, it is also a problem that artificial intelligence cannot solve, as it cannot generate fake data.
Thus, indexing insufficiency is Google’s biggest and real Achilles' heel. Over time, Google will find itself unable to operate without data.
Of course, Google has been trying to address indexing insufficiency.
In 2018, Google launched a cooperation plan with academic databases to access and index these resources through API interfaces. This plan includes cooperation with major academic databases like CrossRef and PubMed, allowing more research papers and academic articles to be searchable by Google.
These efforts have begun to show some results. Through cooperation with PubMed, Google’s search coverage in the medical field has significantly increased. In 2021, Google reported that the accuracy of its medical search results had improved by about 30%, making it easier for users to find the latest medical research and data.
However, this one-by-one access solution is relatively slow and cannot keep up with the pace of new deep web data generation. More critically, much deep web data has no direct value to users and needs to be mined through machine learning, making it difficult for search engines to convey its value to users.
In essence, the problem of indexing insufficiency is not just a technical issue but more likely a systemic issue. Data is gold, so why should I give it to you? What about my data privacy and security? What about my interests? Who can solve these issues?
Blockchain.
4. How Can Blockchain Solve Indexing Insufficiency?
Yes, you read that right. Blockchain can.
Here are two projects using blockchain to solve the problem of deep web data aggregation.
Note, it's about aggregation, not just collection.
The difference is that some data cannot be collected because it is the lifeblood of some companies. Only by providing a mechanism to ensure data security will data owners be willing to share this data for profit; otherwise, it may result in losing both the data and the profits.
Let’s first talk about the deep web data that can be collected.
UpRock is a public data collection project that rewards participants with airdrops.
4.1 UpRock Collects Public Data
UpRock is a decentralized platform using blockchain technology to collect and verify data by sharing unused internet bandwidth.
The general process is as follows:
First, you choose to join the UpRock network. UpRock allows you to turn devices into network nodes by installing an application, similar to renting out idle rooms in the sharing economy.
Then, you execute collection tasks. When your device is idle, it uses the unused bandwidth to connect to the UpRock network. The system automatically assigns data collection tasks, such as scraping product prices, flight information, and online ads.
Finally, data verification ensures accuracy and reliability. Data collected by multiple user devices undergoes cross-verification, ensuring correctness. Think of this process as a group task where everyone checks and confirms the results, ensuring they are accurate.
You might wonder: why can’t Google do this?
4.2 Why Can’t Google Do It?
Because Google’s crawlers are restricted.
Web crawlers are essential tools for traditional search engines like Google to capture public information on the internet. However, crawler programs are limited by website access permissions and the robots.txt protocol, which specifies which pages can be crawled. For example, many financial data sites, academic databases, and specialized platforms restrict access to protect their content, meaning Google may not capture detailed data on these pages.
Websites using robots.txt prevent crawlers from entering critical directories
UpRock uses unused bandwidth from users’ devices to obtain information directly from more data sources. For example, real-time flight data or local market price updates might be captured through devices in those areas sharing bandwidth, which Google finds challenging to obtain in real-time.
Further, the UpRock platform is particularly suited for applications requiring specific and frequent data updates. For example, market analysis companies need real-time product price data and market trends, and ad companies need to monitor the performance and coverage of online ads in real-time. These scenarios demand high data timeliness and accuracy, which traditional search engines struggle to meet, whereas UpRock’s decentralized data collection can provide better support.
The key to ensuring more public data collection is the incentive mechanism.
To encourage participation, UpRock offers a token reward mechanism. Each time you contribute a certain amount of bandwidth or participate in data verification, you earn UpRock tokens ($UPT). If you want to join UpRock, there’s a detailed tutorial available.
Similarly, an application called Grass operates on a similar principle, running in a browser on a computer. There’s also a tutorial for this, which is straightforward and a one-time setup.
Decentralized methods solve public data collection issues, but much valuable deep web data on the internet is still hard to collect. Moreover, this data is of greater value, making data owners highly protective of it.
The blockchain community has a solution called Compute-to-Data. Google has a similar solution called Federated Learning.
4.3 Federated Learning vs. Compute-to-Data
Both technologies are in exploratory stages, so here’s a brief introduction. More details will be provided when significant progress is made.
Compute-to-Data, a technology from the Ocean Protocol project, allows data analysis services to be provided without data leaving the owner's control. In this way, data consumers can perform calculations and analyses without directly accessing or downloading the data itself. This method ensures data privacy while realizing the value of the data.
For example, in the medical field, hospitals and research institutions can share patient data for research without exposing personal privacy information. Similarly, in the financial sector, banks and financial institutions can conduct risk assessments and market analyses without directly sharing sensitive customer data.
Federated Learning, an innovative machine learning method developed by Google, aims to achieve distributed model training while protecting user data privacy. Unlike traditional centralized machine learning methods, Federated Learning distributes the model training process across multiple devices rather than centralizing all data on a server.
Here’s an abstract example: download Google’s mobile keyboard app Gboard, an experimental project for Federated Learning. You’ll experience that as you use it more, Google’s prediction of your next word becomes increasingly accurate.
The general process: after installing Gboard on your phone, it performs personalized model training locally, learning your typing habits and preferences. Every time you input new words or phrases, Gboard updates the language model locally and then sends the updated model to Google’s server. The server aggregates all user model updates, generating a smarter, more accurate global model, which is then distributed to all user devices.
Through this method, Gboard improves typing prediction accuracy while avoiding uploading sensitive user input data to the server, effectively protecting your data privacy and security. (As an aside, if possible, I suggest switching your input method to Gboard. Some domestic input methods can be quite intrusive.)
Clearly, Federated Learning’s application scenarios are primarily in the machine learning field. In contrast, Compute-to-Data provides a more flexible and efficient data processing solution by executing computing tasks at data storage locations. Whether in medical, financial, or market research fields, Compute-to-Data can effectively protect data privacy and ensure efficient data utilization.
However, Compute-to-Data is still in the exploratory stage. The Ocean Protocol market has only 47 Compute-to-Data products, most of which are test cases.
Logically, Compute-to-Data evidently has broader application scenarios than Federated Learning. Yet, until now, we haven’t seen excellent success stories. So, whether blockchain + AI can achieve faster and better deep web data aggregation than web2 giant Google remains uncertain.
Nevertheless, one thing is certain: the incentive mechanism provided by blockchain will undoubtedly be more efficient when combined with AI.
Blockchain’s decentralization and transparent incentive mechanisms can attract more users to participate in data aggregation, enhancing data quality and coverage. The introduction of AI will make these data more effectively analyzed and utilized, further promoting technological progress and application innovation.
Thus, the future direction of technology development will likely be the deep integration of blockchain and AI. This combination is expected to break through current technological limitations, achieving more efficient and secure data processing and analysis, bringing broader application scenarios and commercial value.
5. Google's Innovator’s Dilemma
Incentivizing users to contribute data and participate in search through monetary rewards, like Adot, is bold and effective. However, Google, as the world's largest search engine, has almost no reason to adopt this approach.
First, Google already has a vast user base and rich data resources. Changing the existing business model might introduce unnecessary risks.
Additionally, a strategy of paying users might trigger various complex regulatory and financial issues, which a large company like Google would find challenging to handle.
Moreover, Google can fully leverage AI technology to solve the problem of information overload.
In fact, Google has always been a leader in AI research and application, with robust technical strength and resources. However, if Google were to introduce a search method entirely reliant on AI to organize and arrange information at this stage, it might impact its existing pay-per-click advertising model. This advertising model is Google’s main revenue source. Changing the current search method could weaken advertisers' effectiveness, affecting Google’s income.
Thus, Google would never use AI to solve the problem of information overload, at least not until it finds a new model to replace the current pay-per-click advertising model. Otherwise, it would be akin to severing its relationship with advertisers and sabotaging its revenue stream.
Google is in a classic “innovator’s dilemma,” like a giant ship swaying in a storm.
As the market leader, Google needs to maintain stable growth in existing businesses while continuously innovating to meet emerging competitors' challenges. However, any disruptive innovation might break the existing business model, introducing uncertainty and risk. Thus, in a dilemma, Google often chooses a conservative strategy, waiting for clearer market signals or new business models.
Because of the innovator’s dilemma faced by industry giants like Google, it provides excellent opportunities for newcomers like Adot, UpRock, Grass, and Ocean.
Conclusion
Have you noticed that Adot, UpRock, Grass, and Ocean are all blockchain projects? Do you know why? Because blockchain brings not just advanced productivity but also new production relationships.
In layman's terms, blockchain might not be the best technology to increase the cake’s production, but it is the best to distribute the cake. Traditional search engines and information processing systems mainly focus on faster, more efficient information processing and delivery, while blockchain fundamentally changes information aggregation and value distribution.
Blockchain is essentially a distributed ledger, meaning every participant can transparently see all transactions and data records. On this ledger, the generation, sharing, and use of data are all public and verifiable. This transparency and decentralization give every user more control and participation.
Adot, through its decentralized search network, encourages users to participate in data input and processing and fairly rewards their contributions.
UpRock and Grass allow users with phones or computers to participate easily.
This decentralized model breaks the traditional monopoly structure, giving every participant a chance to share the benefits of the era's development.
In a broader sense, this is not just Google’s dilemma but the era’s dilemma.
Of course, this is also not just an opportunity for newcomers but an opportunity for the entire era.
For more content, please visit the Airdrop Project Base.